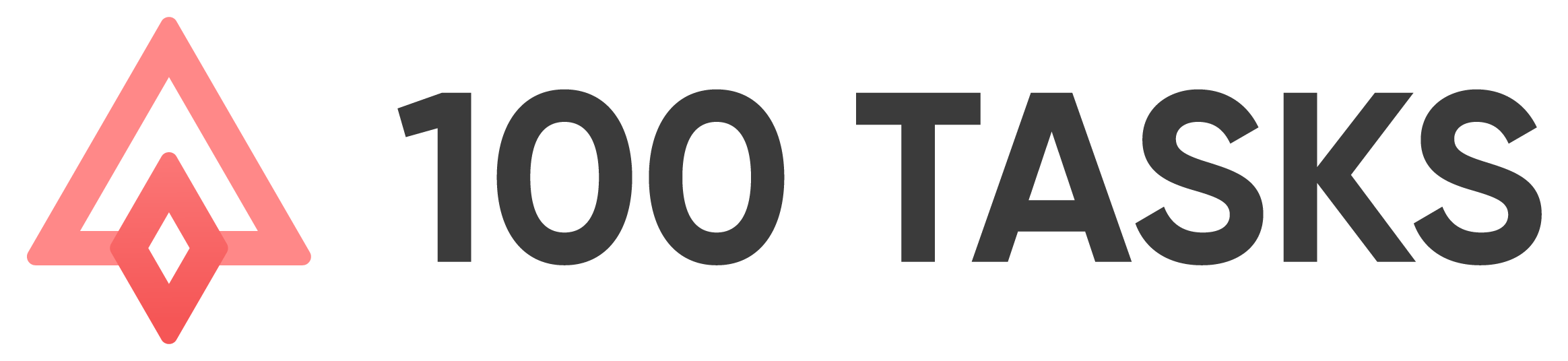
Systematically Build Your Startup. Fast.
Democratizing Entrepreneurship through a Proven Venture Building Process.
Democratizing Entrepreneurship through a Proven Venture Building Process.
100tasks@bell.ventures
Schedule a Demo Call
First off: Can we expect PMs to forecast revenue for new product ideas?
Some time ago, I posted about the importance of financial acumen for product managers.
“Product managers need to consider the financial implications of their decisions. Basic math is a must.
I wouldn't go as saying PMs should 'own the P&L', but they should have the business understanding and strategic insight to create a business case to support their decision-making.
This doesn't need to be highly complex (when we try to predict the future we're usually wrong anyway). Sometimes a rough back-of-napkin calculation is enough to inform decision-making.
For example, to calculate the development cost already invested into a certain client or strategy, you can calculate the story points or actual time spent on that client or strategy (assuming you have neatly assigned the work to an epic or project 🙃 )
To calculate the development effort required for a new feature, take its rough scoping (i.e. roughly 5 sprints) and do the math. If you don't have actual internal labor costs, you can use an approximation of what a developer costs per day to calculate the cost of the scrum team per sprint. (PS don't forget yourself, you - the PM - also cost your company money!)
To calculate projected revenue, make a rough estimation of how this new feature might bring in new revenue (e.g. enabling new sales deals, or expanding existing customers). Stay as close to the truth as you can here (by looking at the actual pipeline & customer database) and don't speculate too much. You can also look at how well past features have performed to grab a baseline.
What followed was an interesting debate about whether it’s even reasonable to expect PMs to predict revenue for ideas - and if so, how it can be done.
Getting to a decent cost calculation is relatively easy. PMs should consider the costs for discovery, delivery (‘cost for one sprint’ benchmark),, and distribution/GTM.
Calculating potential revenue is much, *much* harder, since it depends on a variety of factors outside of our control. The trickiest factor of all: market reception. An awkward fact to this point: appr. 80% of features are hardly or never used.
No matter how hard we try, even with the most robust spreadsheet models and chatGPT by our side, we still can’t predict the future. We’ll have to settle for the best guesstimate we can get by considering the data points we have at our disposal.
In the following section, I distinguish between two main categories:
To keep calculations simple, I’m focusing only on acquisition metrics. When we make product decision x, what new revenue would that unlock (indirectly) or create (directly)? I’m purposefully leaving more complex realities out of scope, such as: what impact will our new product idea have on the retention rates for existing customers?
Examples of high volume, small ticket size business models are B2C or B2B with lower tier customers (e.g. SMEs).
In the section below I outline how you can gather relatively hard data and build forecasts for two example types of product decisions:
Scenario: We’re considering implementing a new feature request based on popular demand from our customers.
“Over the past 6 months, we counted 33 requests for ‘task management features’ from our existing customers. We’re considering adding task management as a separate module at $50/month.”
Firstly: We can’t assume that each of the 33 requesters will buy our task management module once it’s live.
Secondly, for the love of god, don’t pick a market share out of thin air (e.g. Monday.com has 3.31% market share. I guess we can grab 0.5%?!). Just because something works for Monday.com - a $$$ company with strong brand awareness - does not mean it will work for you - a relatively new and unknown startup.
A forecast based on real usage data is far more robust than a forecast based on market data. You have no idea to which extent that market data is transferable to your company or product.
So how to gather that real usage data?
I recommend running either a lightweight fake door test (also called ‘feature stub’ in the product worl) or an MVP test. This allows you to gage actual demand and willingness to pay, so that you can build a forecast based on the basis of that experimentation data.
Include the new task management menu item in your product. Clicking there could lead to a payment gate (‘Buy now’, to capture willingness to pay), after which a ‘coming soon’ message is displayed. Or you can use the space to ask your most burning questions about task management or to allow users to sign up for a co-creation process. The choice is yours!
The main metric you’re interested in is the number of clicks of the button.
Identify the main/highest impact customer job ‘task management’, and design the smallest version of the feature that solves that job. You create a small, yet important part of the product or module. Don’t spend time automating backend processes until you’ve learned that your customers show real interest in your new feature or module.
The main metric you’re interested in is adoption of the MVP. Feature retention (are users coming back to the feature?) can come into play if you’re testing over a longer time horizon.
When choosing your experiment and assessing evidence strength, consider that a high-quality MVP teaches you more than a fake door test - since it shows you actual feature usage, not just button clicks-, but it’s also a lot more expensive and time-consuming to run.
Fake door test results
(number of ‘buy now’ clicks over the time period) / (total traffic over the time period). Adjust the estimated ‘buy now’ clicks taking into account estimated growth in traffic, and factor in drop-off rates in the buying process and customer churn.
MVP test results
50 users have become heavy users of the task management MVP.
80% (leap of faith assumption) of heavy users would be willing to pay. 50 * 80% * $50/month= $2000.
Adjust the estimated revenue taking into account estimated user growth. Factor in drop-off rates in the module buying process and customer churn.
Scenario: Only 10% of our new users are getting to Aha. Of that 10%, 50% upgrade to a paid account.
You ran an A/B test. Your variant included an onboarding survey (“What are you trying to achieve first?”) and sent new users through a personalized onboarding flow. You saw that the number of new users getting to Aha jumped to 40% - whoop whoop! The impact this had on the free-to-paid ratio is significant.
And before you start - You’re totally right: these numbers are not statistically significant! Oh the horror.
Read here to find out why I don’t worry about statistical significance, when we’re merely looking for a directional read to make a reversible product decision.
Forecast the number of signups over the next year, based on sign-up growth from the past months or year. Multiply by 20%. For a full picture: Don’t forget to factor in churn, and adjust by expected macroeconomic / seasonality / any other deviations as needed.
A prime example of a low volume, high ticket size business model is a B2B business selling to enterprise customers. In the section below I outline how you can build a revenue forecast in a typical sales-led scenario: a custom feature request for a new, high-value prospect.
Prospect A - at a predicted ACV of $50,000 - says they will absolutely switch to your product….if only you added Okta Single Sign On to your project management software.
What remains is a painful truth: predicting revenue is impossible. Even when using experimentation basis as a foundation, this is still a far cry from a guarantee on how things will pan out in reality outside of the safe vacuum of an experimentation setting.
Actual revenue growth depends on a wide variety of factors that aren’t just outside your control, but even impossible to comprehend.
Our forecasts will be directionally accurate at best, but never precise.
Because of the above, PMs can’t be held accountable for whether their revenue forecasts have been accurate. If accountability appears to be wildly unfair, then why put effort into building revenue forecasts at all?
I often fall back on Teresa Torres’ distinction between business and product metrics:
Business metrics (such as revenue growth or customer churn) lag behind a change in customer behavior or sentiment. Staring at business metrics leaves Product Managers without a ‘so what’. Seeing a dip or increase in revenue leads to reactive work (trying to figure out what caused it), but hardly ever leads to actionable insights.
Product metrics measure whether your customers are likely getting value from your product, which is made visible through a change in their behavior or sentiment. For example: more users are getting to Aha, meaning that they experience the core value of your product for the first time. An increase in your sign-up-to-Aha rate is detected far more quickly than an increase in revenue (lagging indicator!). This product metric is also far better to inspire action, for example by looking at what might be blocking users from experiencing their Aha.
Product metrics are traditionally set by the product team in close collaboration with Leadership. Leadership should ensure that the product metric has a high likelihood of driving the primary business metric. It’s good to create customer value, it’s better if that also has a positive impact on your bottom line.
Rather than asking your Product Manager to create a revenue growth forecast, ask them to predict the outcome on their product metric. This is the realm where they should ‘play’.
It’s essential to close the loop: You need to check whether the positive impact on your team’s product metric moved the needle on the business metric (e.g. revenue).
The big issue with revenue forecasts is that we’re at risk of sinking a lot of time into them, without getting much value. We can spend weeks building a beautiful forecast in Excel, and find out a year later that we were completely off.
Some voices plead for dropping the charade and using ICE instead, where impact is a ballpark revenue or margin figure and effort is a count of estimated developer+design weeks. This method attempts to quantify the potential impact in financial terms rather than using less precise sizing methods.
The problem of the inaccurate Impact estimate remains, but at least we steer clear from going down the forecasting rabbit hole, and we can be more honest about how rough our estimate is.
Accurately forecasting revenue for product ideas is a challenge, to say the least. It involves using proxies and estimates that are directionally accurate, rather than precise. Pulling in historical data, market data, and experimentation data can make forecasts more precise, but the harsh reality remains: We can’t predict the future.
It’s essential to measure the accuracy of our revenue forecasts after launch so that we can improve our forecasting skills - or do away with revenue forecasts altogether. A forecast is nice, but measurable outcomes are what matters.
I'm an interim product lead, product advisor & product coach. I help product teams at B2B SaaS startups & scale-ups move toward outcome-driven and experimentative ways of working. Customer discovery and rapid experimentation are my jam.
- Read Else van der Berg's insightful blog article about the Importance of Financial Acumen for Product Managers on LinkedIn for deeper insights into product management and financial acumen.
Martin Bell (Founder & CEO of Bell Ventures) is the visionary and driving force behind the hyper-successful 100 Tasks Startup System which has driven the growth of 20,000+ startups including Zalando and Delivery Hero.
At Rocket Internet, he pioneered the 100-Day-Launch process and led 120+ private and public sector venture-building projects.
Now Martin aims to democratize entrepreneurship by sharing his invaluable practical knowledge and tools to empower aspiring entrepreneurs just like you. Does that sound like you? Then make sure to learn more below ...